
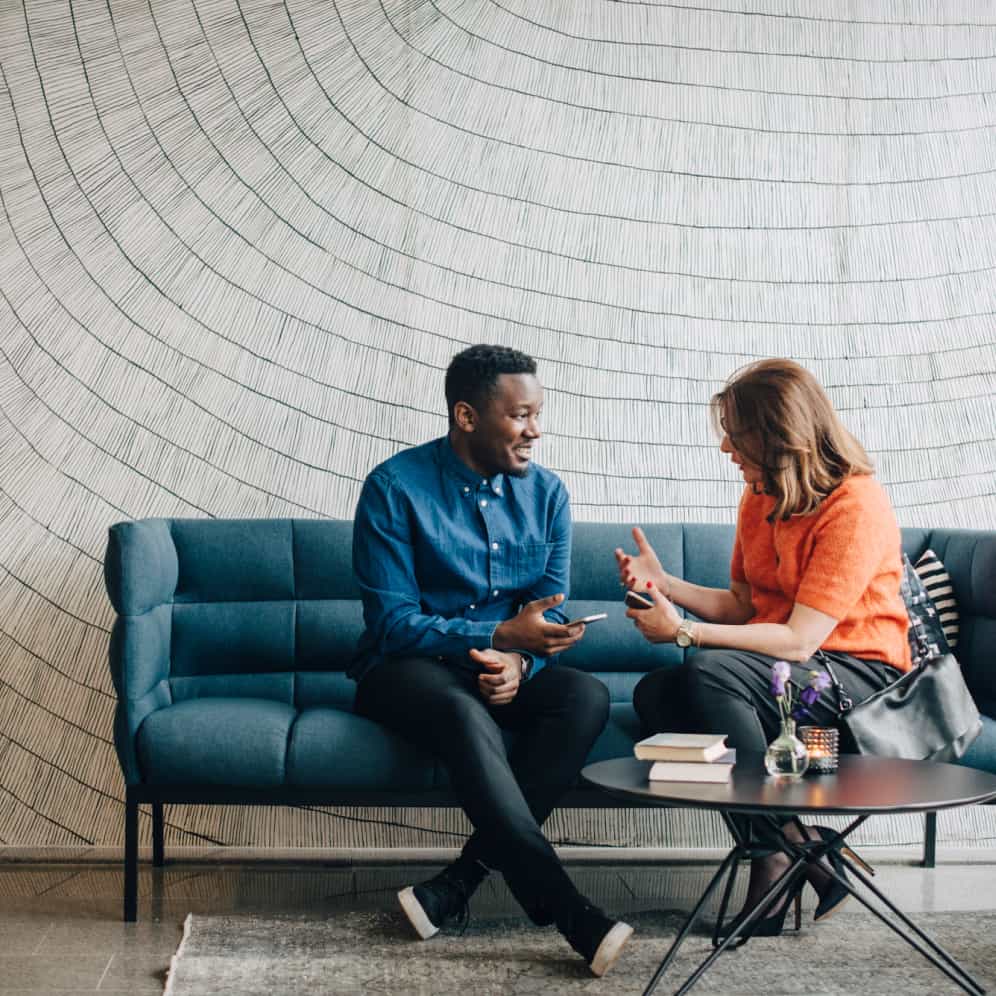
settings_input_component
Unique needs, powerful solutions
In today’s complex business world, we know one size doesn't fit all. That's why we offer tailored solutions to your specific needs.
switch_access_shortcut
Extended reach
Trusted partner companies around the world extend Teradata's reach into new geographies, industries, and specialties.
Find a Teradata partner
A broad ecosystem of partners to provide you with the best data and analytics solutions
Related